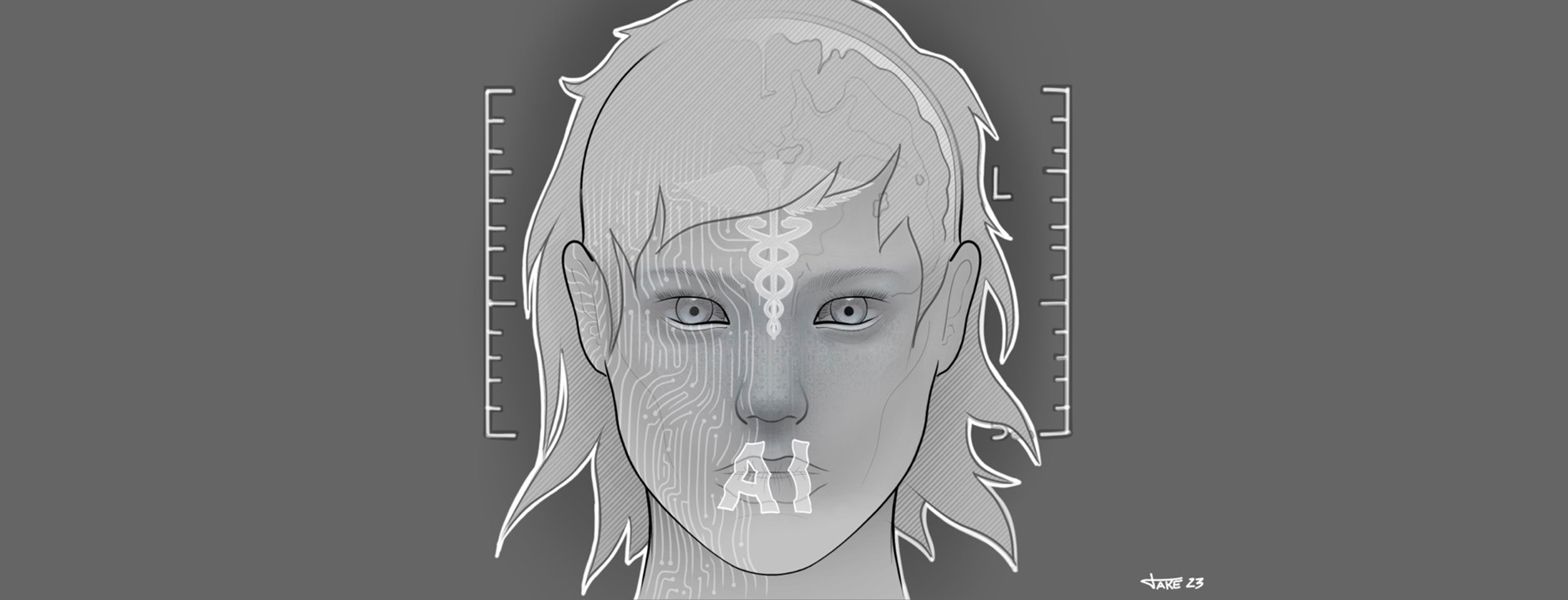
What is artificial intelligence (AI)? Is it the evoking computer from sci-fi aware of its own existence and determined to destroy humanity? Is it a robot that does our job for us while we kick our feet up? Right now, maybe it is neither, it can be defined as “A System that mimics human intelligence to perform complex tasks using advanced learning algorithms that capture underlying patterns and relationships from the data they collect.” The tasks and benefits from such a system can be many but generally serve as three main use categories: accuracy improvement, automation of tasks, or a recommendations engine.
In developing a SAMD (Software As a Medical Device) product consider both the regulatory guidelines and best practices. The FDA is partnering with industry to develop regulations in this emerging field, they recently released a guidance on Clinical Decision Support Software describing the criterion in which software is considered a medical device by the agency. And, during software life-cycle development, ISO 62304 outlines the processes of risk management, maintenance, configuration management, and problem resolution.
Developers should build in systems on the front end for data mining whether in the form of document capturing tools, video data collection, speech recognition, or otherwise. And, comprehensive cybersecurity around these data sources in addition to the access, analysis, and output systems.
Lastly, algorithms should take bias into account. This is already present in the diagnosis making process today, clinicians can jump to conclusions based on early information and stick to their guns even as new information becomes available (premature closure / anchoring). The algorithms themselves can have bias, in how data is fitted when machine learning is automated.
- Automation Bias: Tendency of people to show deference to automated output, maybe due to person’s lack of confidence/experience, or assumption that the automation designed to make the correct determination.
- Fitting Bias: Over Fitting- Automation has been overly relying on the trained data and does not provide correct responses when given new information. Under Fitting - Machine is under trained and doesn’t correctly identify relationships between the variables.
Widespread AI use is in its infancy, its currently being leveraged across several surgical products currently on the market including surgery planners, guidance systems, AR, blood loss monitoring, and predictive analytics. The future holds many opportunities for AI to burn down existing healthcare challenges.
Accuracy Improvement:
- Comprehensive Patient Medical Information
- Summarization and Highlighting of Patient Case History
- Accurate Encoding of procedures and diagnosis for insurance
- Accurate diagnosis from medical images
- Risk-aware decision making –using predictive analysis of surgical outcome, implant choice, length of hospital stay, risk of re-hospitalization
- Post op x-ray, feedback loop, feedback to surgeon on trending accuracy stats, predictive risks
- Physician burnout - make less errors during diagnosis
- Physician shortage – making fewer surgeons more efficient
Automation Enabled Improvements:
- Improved surgical planning / operation
- AI-assisted surgical robotics
- Supply chain automation
- Reduced non-conformances, out-of-commission instrument sets
- Reduced waste, reprocessing costs
- Smart intra-op assistant / training
Recommendations Engine:
- Patient/procedure/surgeon customized device on demand
- Fair surgeon success ratings based on predictive risk/outcomes
- Informing consumers on surgeon/facility for their condition to maximize outcomes
It probably won’t be too far into the future before some of these AI-enabled improvements become mainstream practice in the healthcare domain. The recent advances in ChatGPT have shown how complex knowledge intensive tasks such as text summarization, essay generation, intelligent Q&A (Question and Answer), etc. can be accomplished by current language models. Convolutional Neural Networks (CNN)-based deep learning models are showing promise for automatic detection and classification of tumors in medical imaging. Advanced Machine Learning (ML), Rule-based modeling, and Embedded-AI can help with addressing other opportunities such as risk prediction, improved surgical planning, AI-assisted robotic devices, supply chain automation, and customized recommendations
AI will help in bringing consistency in the process, improve overall efficiency, reduce cost of operations while adhering and improving the regulatory compliance.
Interested in implementing AI/ML technology into your business?
Kaleidoscope uses advanced learning algorithms to capture patterns and relationships within your data to help you better understand the data collected and provide both exploratory and predictive analytics based on findings. Contact Matt Suits: [email protected]