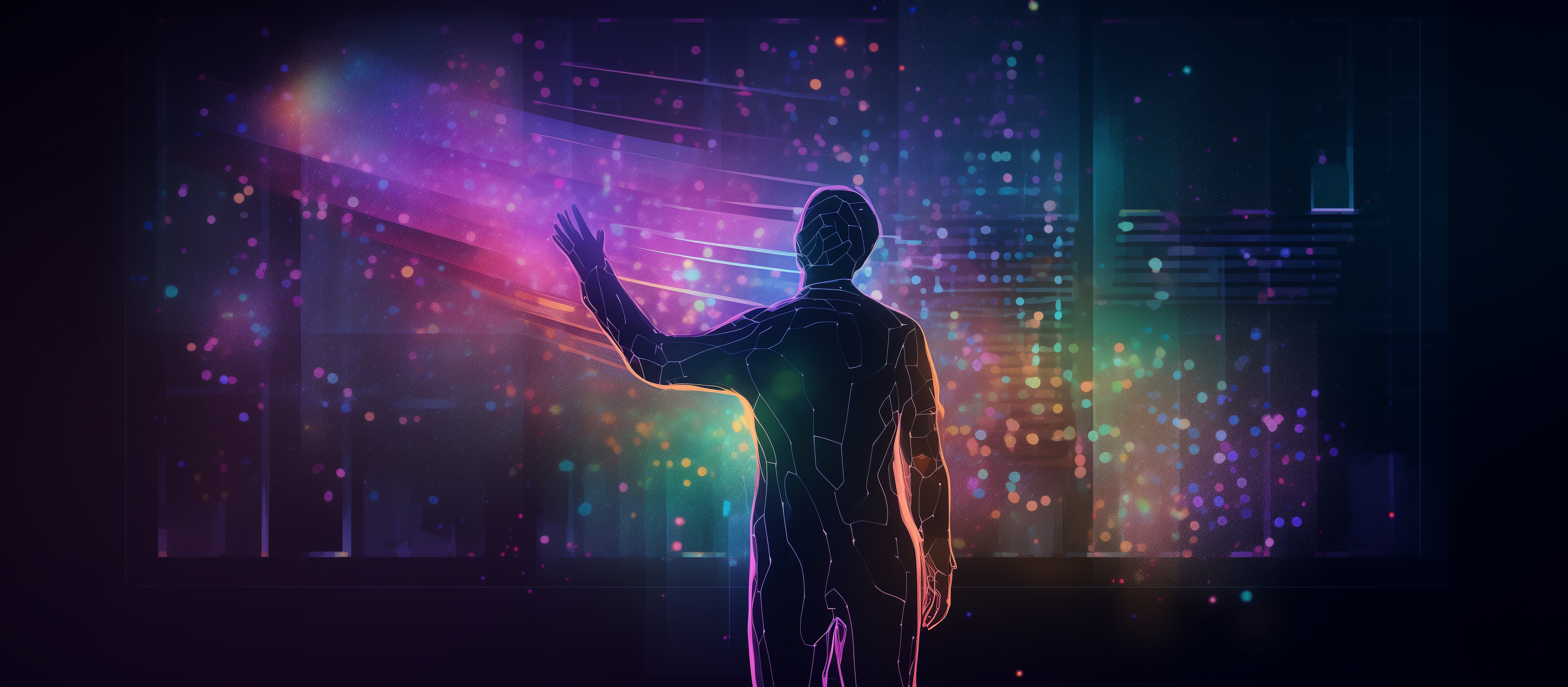
BACKGROUND
Qualitative research plays a pivotal role in enriching our comprehension of individual narratives and experiences. It is a cornerstone methodology for design researchers seeking to forge a deep connection with user perspectives, particularly during the initial phases of the design process. This approach is instrumental in guiding iterative design developments, ensuring that end-user needs are comprehensively addressed. Qualitative data encompasses a diverse array of formats, including textual content, photographs, and videos. Typically, these studies involve a more focused sample size, often with 10 or fewer participants, to facilitate an intensive, detail-oriented analysis that quantitative methods may not capture.
Although qualitative research is the methodology of choice for design researchers, the approach requires a considerable time commitment. Qualitative data is known for being unwieldy at times, and words and images require more hours of analysis than numeric data. Often, our clients are eager to obtain research findings as quickly as possible to move a product or system into production. Therefore, large scale qualitative studies are not feasible for most design research projects. With the recent surge in the availability of AI language model tools, we speculated that ChatGPT could be used to analyze extremely large sets of qualitative data more efficiently. To that end, we conducted a 6-month project testing ChatGPT as a potential tool for qualitative data analysis.
THE CURRENT PROJECT
Our aim in conducting this project was to determine if AI could produce insights from a large dataset that would otherwise be unmanageable and time prohibitive for a human researcher. We used data from 25,000 open response questions to explore the capacity and capability of ChatGPT as computer-assisted qualitative data analysis software (CAQDAS). The dataset we used was provided by the VIA Institute on Character, a local non-profit organization with which we are affiliated. We decided to experiment with ChatGPT to determine if it could reliably and accurately analyze text data. Our expectation was that if ChatGPT could analyze qualitative datasets with tens or hundreds of thousands of respondents, new pathways for qualitative researchers may develop.Using AI for data analysis could change the trajectory of a research design and lead to large scale qualitative studies that were not possible before now.
PROCEDURE
To test the limits of ChatGPT 4.0 (the only version with the means to upload files), we tried two different approaches to determine the capability of the tool.
METHOD 1: QUICK AND EASY
We started with a vague set of user queries to place the data preparation load on the CAQDAS and to determine if it would complete the same tasks a human researcher would.
User Query: Analyze the data in column AQ, identify themes, and provide 3-5 insights based on participant responses.
Result: Not useful.
- ChatGPT did not automatically clean the data without instruction which caused an error. The output from ChatGPT indicated the data file was either too long or too complex and it was unable to proceed with analysis. The raw data included responses such as “N/A” or random strings of letters, which a researcher would have deleted or ignored before analysis.
- Lacking more specific instruction, ChatGPT defaulted to a quantitative approach to data analysis, even though the data were text responses. One of the first outputs ChatGPT produced was a count of the most common phrases in the dataset.
METHOD 2: THE GUIDED ANALYST
We then provided ChatGPT with more specific instructions. We instructed it toclean, review and code the data, then create insights using a theoretical framework as a guide for analysis.
User Query: I'd like to analyze some text data using Peace Psychology and Positive Psychology as theoretical frameworks. Include content from the VIA Institute on Character as an additional framework. Focus on data in the 'Open Responses_Political Differences' column.
First, ignore text that indicates a respondent did not want to answer such as 'N/A' or random strings of letters. Leave those cells blank. Next, use descriptive codessuch as a phrase that describes the content of the targeted data.
Create a new document and filter the data from columnAD. Group the data according to the codes created in columnAD and list each data point that corresponds to the code.
Create 3-5 insights using the coded data in ColumnAD using positive psychology and peace psychology as theoretical frameworks.
Create a Word document and place the insights you created in it. Make the file available for download.
Result: Success
ChatGPT produced a list of five insights using the VIA classification of character strengths as a framework, supported by references to positive psychology and peace psychology.
Our last step was to think about how to test the accuracy and reliability of the findings. Rachael has a strong background in qualitative methodology, so she extracted a sample of 20 data points and used a traditional qualitative approach to code the data. We also queried ChatGPT using the same data set with similar user queries to determine the degree of similarity between both sets of outputs.
Accuracy
Within the first 20 rows of participant responses, we identified at least three codes related to the five insights ChatGPT produced. We were pleased with the accuracy of the codes as they related to the insights from our sample data.
Reliability
Both researchers requested insight generation from ChatGPT using similar but not identical queries. Chat GPT’s output for both sets of queries was closely aligned. Three of the five generated insights were nearly identical between the two researchers and two were unique per researcher. It is important to note that even within research teams, differences arise between researchers’ interpretation of qualitative data. Disagreements are resolved through discussion and are typical of a qualitative research project.
Finally, we tested some of the limits of ChatGPT with several ad hoc user queries. Ourqueries produced a great deal of information in a very short period.
AD HOC REQUESTS:
- Code Participant Country of Origin: We queried the data set to determine if each participants’ country of origin represented an individualistic or collectivist nation(coded as “country designation”). We asked ChatGPT to code each participant’s country of origin using this designation. The codes were placed in a new column created by ChatGPT.
- Group Data and Analyze Frequency: We asked ChatGPT to examine the most common keywords and themes by country of origin. It provided a summary for all countries represented in this data set with the most frequently used codes according to participant’s country of origin.
- Character Strength Frequency by Country Designation: We prompted ChatGPT to organize the data according to country designation and create a bar chart to illustrate the frequency of character strengths chosen as most beneficial by participants.
All the ad hoc requests were completed successfully. ChatGPT confirmed its approach with users before completing each step. For example, after requesting the data be coded according to country designation, ChatGPT responded:
Sure! In order to label countries as "collectivist" or "individualist," I would need a reference or criteria to determine which category each country falls into. There are various sources and studies that have categorized countries based on their cultural dimensions, including the work by Geert Hofstede.
Do you have a specific reference or criteria in mind that you'd like me to use? Alternatively, I can use Hofstede's cultural dimensions as a general guideline.
ChatGPT also encouraged us to use caution when interpreting the findings from the between-country analysis, because significantly more participants were from individualistic nations.
LESSONS LEARNED
We learned through this project that ChatGPT exhibits several esoteric preferences for working with Excel files. We only used Excel to upload data sets, so our suggestions are restricted to this software.
1. ChatGPT cannot analyze data if it has been tagged with a data type. The output will state that it completed the user query, but new files will not show any changes.
SOLUTION: Remove any Data Types tags before uploading Excel files toChatGPT.
2. ChatGPT prefers references to column names instead of the letters Excel uses to identify columns.
SOLUTION: If a user query contains a letter identifier instead of a column name, remove the space between the word “Column” and the letter.
CORRECT: “Provide a mean for the data in columnAI.”
INCORRECT: “Provide a mean for the data in Column AI.”
3. Unless instructed, ChatGPT will not automatically clean uploaded data. If a user attempts to request analysis before cleaning, it will respond with an error message.
SOLUTION: Provide explicit instructions for data cleaning before analysis.
HUMAN RESEARCHER VALUE
We shared just a fraction of the user queries we submitted over a 6-month period to test ChatGPT as a qualitative analysis tool. We presented the successes and failuresas linear, concise processes for readability. However, early in the project, ChatGPT was often overwhelmed with requests and our queries resulted in error messages. Queries usually required several back-and-forth inputs between researchers and the AI to clarify instructions. With little or no guidance, ChatGPT was unable to produce results. We found that the AI required specific instructions to function as computer-assisted qualitative data analysis software. Our bottom-line recommendation is that well trained researchers test the tool using a data set for which they already possess human produced findings. Compare those findings with ChatGPT's output and evaluate its reliability and accuracy.
Based on our brief examination of ChatGPT’s capability, we advise only well-trained researchers with extensive qualitative research experience to use AI as a computer-assisted data analysis tool. As in any other profession, expertise and training are the best predictors of quality work. As the saying goes, garbage in garbage out.Users with no idea how to design a rigorous research study will not provide the needed input for AI to perform adequately.
Our early work indicates the potential for AI to assist in qualitative data analysis. Like other CAQDAS products such as MAXQDA and NVivo, the software serves as a management and organizational tool. We envision ChatGPT as a marginally higher-leveltool with the capacity for categorizing and summarizing qualitative data, with the proper guidance and instruction.